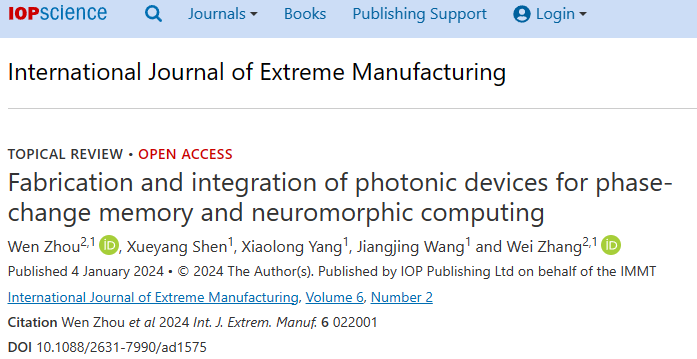
In the past decade, there has been tremendous progress in integrating chalcogenide phase-change materials (PCMs) on the silicon photonic platform for non-volatile memory to neuromorphic in-memory computing applications. In particular, these non von Neumann computational elements and systems benefit from mass manufacturing of silicon photonic integrated circuits (PICs) on 8-inch wafers using a 130 nm complementary metal-oxide semiconductor line. Chip manufacturing based on deep-ultraviolet lithography and electron-beam lithography enables rapid prototyping of PICs, which can be integrated with high-quality PCMs based on the wafer-scale sputtering technique as a back-end-of-line process. In this article, we present an overview of recent advances in waveguide integrated PCM memory cells, functional devices, and neuromorphic systems, with an emphasis on fabrication and integration processes to attain state-of-the-art device performance. After a short overview of PCM based photonic devices, we discuss the materials properties of the functional layer as well as the progress on the light guiding layer, namely, the silicon and germanium waveguide platforms. Next, we discuss the cleanroom fabrication flow of waveguide devices integrated with thin films and nanowires, silicon waveguides and plasmonic microheaters for the electrothermal switching of PCMs and mixed-mode operation. Finally, the fabrication of photonic and photonic–electronic neuromorphic computing systems is reviewed. These systems consist of arrays of PCM memory elements for associative learning, matrix-vector multiplication, and pattern recognition. With large-scale integration, the neuromorphicphotonic computing paradigm holds the promise to outperform digital electronic accelerators by taking the advantages of ultra-high bandwidth, high speed, and energy-efficient operation in running machine learning algorithms.
Link:https://iopscience.iop.org/article/10.1088/2631-7990/ad1575