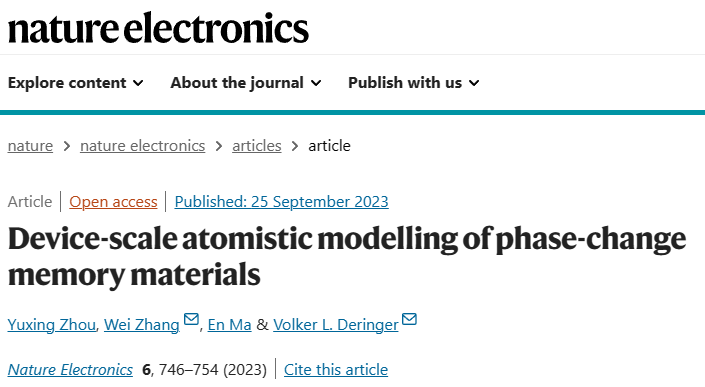
Computer simulations can play a central role in the understanding of phase-change materials and the development of advanced memory technologies. However, direct quantum-mechanical simulations are limited to simplified models containing a few hundred or thousand atoms. Here we report a machine-learning-based potential model that is trained using quantum-mechanical data and can be used to simulate a range of germanium–antimony–tellurium compositions—typical phase-change materials—under realistic device conditions. The speed of our model enables atomistic simulations of multiple thermal cycles and delicate operations for neuro-inspired computing, specifically cumulative SET and iterative RESET. A device-scale (40 × 20 × 20 nm3) model containing over half a million atoms shows that our machine-learning approach can directly describe technologically relevant processes in memory devices based on phase-change materials.
Link:Device-scale atomistic modelling of phase-change memory materials | Nature Electronics