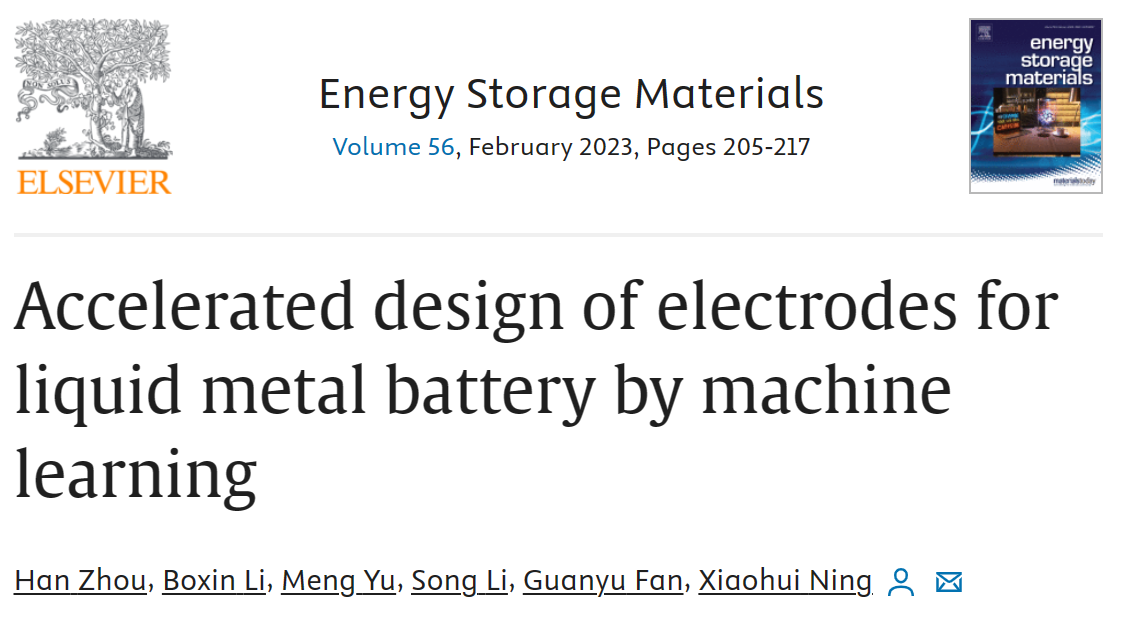
The electrochemical performance of liquid metal batteries (LMBs) mainly relies on the rational design of electrode materials. Nevertheless, the traditional ways of optimizing electrode materials via trial-and-error methods consume much time and effort. Herein, we present an experimentally validated machine learning approach with a self-correction function to expedite the discovery of novel multi-element electrodes with optimal electrochemical properties for LMBs, where the alloy-related, LMB-related, and creative features are established as effective input parameters. LMBs dataset, augmented from 814 original data to 2158 efficacious data, successfully predicts the quaternary alloy electrodes with discharge voltage, discharge energy and energy density. Four screened alloy electrodes are successfully synthesized and confirmed to have superior performance. Experimental results reveal the as-designed LMBs show high coulombic efficiency (∼99%), high energy efficiency (∼87%), high energy density (∼280 Wh kg−1), and low energy cost (∼70 $ kWh−1), achieving good agreement with the predictions. This method opens up exciting new possibilities for the speedy screening of optimal electrode materials for maximizing LMBs performance.
Link:Accelerated design of electrodes for liquid metal battery by machine learning - ScienceDirect